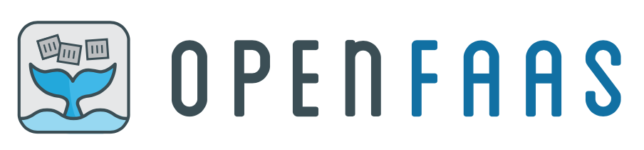
This is the fourth post in this series. The focus will be on getting OpenFaaS set up on your Raspberry Pi Kubernetes cluster nice and quickly.
Here are some links to previous posts in this series:
- Building a Raspberry Pi Kubernetes Cluster – Part 1 – Routing
- Building a Raspberry Pi Kubernetes Cluster – Part 2 – Master Node
- Building a Raspberry Pi Kubernetes Cluster – Part 3 – Worker Nodes and MetalLB
OpenFaaS is an open source project that provides a scalable platform to easily deploy event-driven functions and microservices.
It has great support to run on ARM hardware, which makes it an excellent fit for the Raspberry Pi. It’s worth mentioning that it is of course designed to run across a multitude of different platforms other than the Pi.
Getting Started
You’ll work with a couple of different CLI tools that I chose for the speed at which they can get you up and running:
- faas-cli – the main CLI for OpenFaaS
- arkade – a golang based CLI tool for quick and easy one liner installs for various apps / software for Kubernetes
There are other options like Helm or standard YAML files for Kubernetes that you could also use. Find more information about these here.
I have a general purpose admin and routing dedicated Pi in my Raspberry Pi stack that I use for doing admin tasks in my cluster. This made for a great bastion host that I could use to run the following commands:
Install arkade
# Important! Before running these scripts, always inspect the remote content first, especially as they're piped into sh with 'sudo' # MacOS or Linux curl -SLsf https://dl.get-arkade.dev/ | sudo sh # Windows using Bash (e.g. WSL or Git Bash) curl -SLsf https://dl.get-arkade.dev/ | sh
Install faas-cli
# Important! Before running these scripts, always inspect the remote content first, especially as they're piped into sh with 'sudo' # MacOS brew install faas-cli # Using curl curl -sL https://cli.openfaas.com | sudo sh
Deploying OpenFaaS
Using arkade, deploy OpenFaaS with:
arkade install openfaas
If you followed my previous articles in this series to set your cluster up, then you’ll have a LoadBalancer
service type available via MetalLB. However, in my case (with the above command), I did not deploy a LoadBalancer
service, as I already use a single Ingress Controller for external traffic coming into my cluster.
The assumption is that you have an Ingress Controller setup for the remainder of the steps. However, you can get by without one, accessing OpenFaaS by the external gateway NodePort
service instead.
The arkade install will output a command to get your password. By default OpenFaaS comes with Basic Authentication. You’ll fetch the admin password you can use to access the system with Basic Auth next.
Grab the generated admin password and login with faas-cli
:
PASSWORD=$(kubectl get secret -n openfaas basic-auth -o jsonpath="{.data.basic-auth-password}" | base64 --decode; echo) echo -n $PASSWORD | faas-cli login --username admin --password-stdin
OpenFaaS Gateway Ingress
OpenFaaS will have deployed with two Gateway services in the openfaas namespace.
- gateway (ClusterIP)
- gateway-external (NodePort)
Instead of relying on the NodePort service, I chose to create an Ingress Rule to send traffic from my cluster’s Ingress Controller to OpenFaaS’ ClusterIP service (gateway).
You’ll want SSL so setup a K8s secret to hold your certificate details for the hostname you choose for your Ingress Rule. Here is a template you can use for your OpenFaaS ingress:
apiVersion: extensions/v1beta1 kind: Ingress metadata: annotations: kubernetes.io/ingress.class: nginx nginx.ingress.kubernetes.io/rewrite-target: / name: openfaas spec: rules: - host: openfaas.foo.bar http: paths: - backend: serviceName: gateway servicePort: 8080 path: / tls: - hosts: - openfaas.foo.bar secretName: openfaas.foo.bar
Create your TLS K8s secret in the openfaas namespace, and then deploy the ingress rule with:
kubectl -n openfaas apply -f ./the_above_ingress_rule.yml
You should now be able to access the OpenFaaS UI with something like https://openfaas.foo.bar/ui/
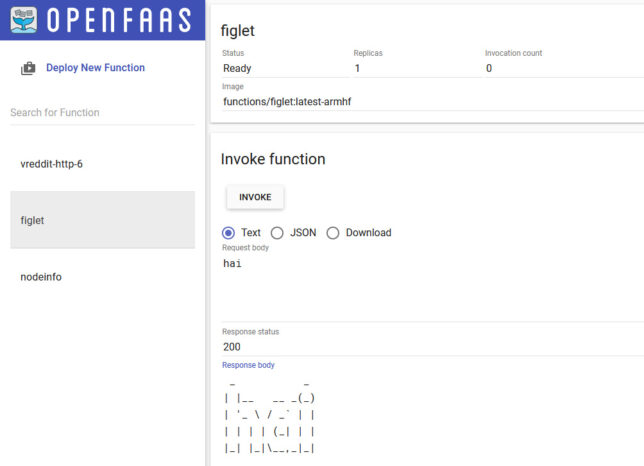
Creating your own Functions
Life is far more fun on the CLI, so get started with some basics with first:
faas-cli store list --platform armhf
– show some basic functions available for armhf (Pi)faas-cli store deploy figlet --platform armhf
– deploy the figlet function that converts text to ASCII representations of that textecho "hai" | faas-cli invoke figlet
– pipe the text ‘hai’ into the faas-cli invoke command to invoke the figlet function and get it to generate the equivalent in ASCII text.
Now, create your own function using one of the many templates available. You’ll be using the incubator template for python3 HTTP. This includes a newer function watchdog (more about that below), which gives more control over the HTTP / event lifecycle in your functions.
Grab the python3 HTTP template for armhf and create a new function with it:
# Grab incubator templates for Python, including Python HTTP. Will figure out it needs the armhf ones based on your architecture! faas template pull https://github.com/openfaas-incubator/python-flask-template faas-cli new --lang python3-http-armhf your-function-name-here
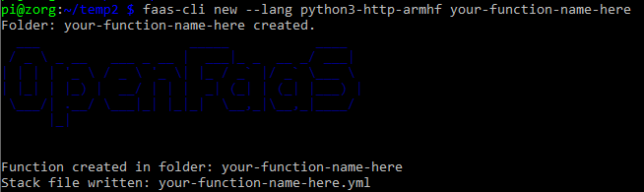
A basic file structure gets scaffolded out. It contains a YAML file with configuration about your function. E.g.
version: 1.0 provider: name: openfaas gateway: http://127.0.0.1:8080 functions: your-function-name-here: lang: python3-http-armhf handler: ./your-function-name-here image: your-function-name-here:latest
The YAML informs building and deploying of your function.
A folder with your function handler code is also created alongside the YAML. For python it contains handler.py
and requirements.txt
(for python library requirements)
def handle(event, context): # TODO implement return { "statusCode": 200, "body": "Hello from OpenFaaS!" }
As you used the newer function templates with the latest OF Watchdog, you get full access to the event and context in your handler without any extra work. Nice!
Build and Deploy your Custom Function
Run the faas up
command to build and publish your function. This will do a docker build
/ tag
/ push
to a registry of your choice and then deploy the function to OpenFaaS. Update your your-function-name-here.yml
file to specify your desired docker registry/repo/tag, and OpenFaas gateway address first though.
faas up -f your-function-name-here.yml
Now you’re good to go. Execute your function by doing a GET request to the function URL, using faas invoke
, or by using the OpenFaaS UI!
Creating your own OpenFaaS Docker images
You can convert most Docker images to run on OpenFaaS by adding the function watchdog to your image. This is a very small HTTP server written in Golang.
It becomes the entrypoint which forwards HTTP requests to your target process via STDIN or HTTP. The response goes back to the requester by STDOUT or HTTP.
Read and find out more at these URLs:
Hopefully this gave you a good base to get started with OpenFaaS. We covered everything from deployment and configuration, to creating your own custom functions and images. Have fun experimenting!