Most of the steps in this how to post can be applied to any Kubernetes cluster to get an NGINX Ingress Controller deployed, so you don’t necessarily have to be running Kubernetes in DigitalOcean. With that said, let’s go through the process of setting up a Kubernetes cluster with NGINX Ingress Controller on DigitalOcean.
DigitalOcean have just officially announced their own Kubernetes offering so this guide covers initial deployment of a basic worker node pool on DigitalOcean, and then moves on to deploying an Ingress Controller setup.
If you’re thinking of signing up on DigitalOcean, consider using my referral link below. It’ll net you $100 of credit to spend over 60 days, and if you stick with them I’ll get a small $25 credit to my own account. Win win!
My Referral link to sign up with DigitalOcean
Note: If you already have a Kubernetes cluster setup and configured, then you can skip the initial cluster and node pool provisioning step below and move on to the Helm setup part.
Deploy a Kubernetes node pool on DigitalOcean
You could simply do this with the Web UI console (which makes things really simple), but here I’ll be providing the doctl commands to do this via the command line.
First of all, if you don’t have it already download and setup the latest doctl release. Make sure it’s available in your PATH.
Initialise / authenticate doctl. Provide your own API key when prompted.
doctl auth init
Right now, the help documentation in doctl version 1.12.2 does not display the kubernetes related commands arguments, but they’re available and do work.
Create a new Kubernetes cluster with just a single node of the smallest size (you can adjust this to your liking of course). I want a nice cheap cluster with a single node for now.
doctl k8s cluster create example-cluster --count=1 --size=s-1vcpu-2gb
The command above will provision a new cluster with a default node pool in the NYC region and wait for the process to finish before completing. It’ll also update your kubeconfig file if it detects one on your system.

Once it completes, it’ll return and you’ll see the ID of your new cluster along with some other details output to the screen.
Viewing the Kubernetes console in your browser should also show it ready to go. You can download the config from the web console too if you wish.
Kubeconfig setup
If you’re new to configuring kubectl to manage Kubernetes, follow the guide here to use your kube config file that DigitalOcean provides you with.

Handling different cluster contexts
With kubectl configured, test that it works. Make sure you’re in your new cluster’s context.
kubectl config use-context do-nyc1-example-cluster
If you’re on a Windows machine and use PowerShell and have multiple Kubernetes clusters, here is a simple set of functions I usually add to my PowerShell profile – one for each cluster context that allows easy switching of contexts without having to type out the full kubectl command each time:
Open your PowerShell profile with:
notepad $profile
Add the following (one for each context you want) – make sure you replace the context names with your own cluster names:
function kubecontext-minikube { kubectl config use-context minikube }
function kubecontext-seank8s { kubectl config use-context sean.k8s.local }
function kubecontext-digitalocean { kubectl config use-context do-nyc1-example-cluster }
Simply enter the function name and hit enter in your PS session to switch contexts.
If you didn’t have any prior clusters setup in your kubeconfig file, you should just have your new DigitalOcean cluster context selected already by default.
Deploy Helm to your cluster
Time to setup Helm. Follow this guide to install and configure helm using kubectl.
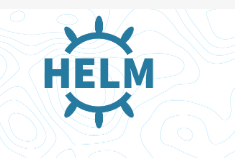
Deploy the Helm nginx-ingress chart to enable an Ingress Controller on DigitalOcean in your Kubernetes cluster
Now that you have helm setup, you can easily deploy an Ingress Controller to your cluster using the nginx helm chart (package).
helm install --name nginx-ingress stable/nginx-ingress --set service.type=LoadBalancer --namespace default
When you specify the service.type of “LoadBalancer”, DigitalOcean will provision a LoadBalancer that fronts this Kubernetes service on your cluster. After a few moments the Helm deployment should complete (it’ll run async in the background).
You can monitor the progress of the service setup in your cluster with the following command:
kubectl --namespace default get services -o wide -w nginx-ingress-controller
Open the Web console, go to Networking, and then look for Load Balancers.
You should see your new NGINX load balancer. This will direct any traffic through to your worker pool node(s) and into the Kubernetes Service resource that fronts the pods running NGINX Ingress.

At this point you should be able to hit the IP Address in your web browser and get the default nginx backend for ingress (with a 404 response). E.g.

Great! This means it’s all working so far.
Create a couple of basic web deployments inside your cluster
Next up you’ll create a couple of very simple web server Deployments running in single pods in your cluster’s node pool.
Issue the following kubectl command to create two simple web deployments using Google’s official GCR hello-app image. You’ll end up with two deployments and two pods running separately hosted “hello-app” web apps.
kubectl run web-example1 --image=gcr.io/google-samples/hello-app:2.0 --port=8080
kubectl run web-example2 --image=gcr.io/google-samples/hello-app:2.0 --port=8080
Confirm they’re up and running wth 1 pod each:
kubectl get deployments
NAME DESIRED CURRENT UP-TO-DATE AVAILABLE AGE
web-example1 1 1 1 1 12m
web-example2 1 1 1 1 23m
Now you need a service to back the new deployment’s pods. Expose each deployment with a simple NodePort service on port 8080:
kubectl expose deployment/web-example1 --type="NodePort" --port 8080
kubectl expose deployment/web-example2 --type="NodePort" --port 8080
A NodePort service will effectively assign a port number from your cluster’s service node port range (default between 30000 and 32767) and each node in your cluster will proxy that specific port into your Service on the port you specify. Nodes are not available externally by default and so creating a NodePort service does not expose your service externally either.
Check the services are up and running and have node ports assigned:
kubectl get services
NAME TYPE CLUSTER-IP EXTERNAL-IP PORT(S) AGE
web-example1 NodePort 10.245.125.151 <none> 8080:30697/TCP 13m
web-example2 NodePort 10.245.198.91 <none> 8080:31812/TCP 24m
DNS pointing to your Load Balancer
Next you’ll want to set up a DNS record to point to your NGINX Ingress Controller Load Balancer IP address. Grab the IP address from the new Kubernetes provisioned Load Balancer for Ingress from the DigitalOcean web console.
Create an A record to point to this IP address.
Create your Ingress Rules
With DNS setup, create a new YAML file called fanout.yaml:
This specification will create an Kubernetes Ingress Resource which your Ingress Controller will use to determine how to route incoming HTTP requests to your Ingress Controller Load Balancer.
apiVersion: extensions/v1beta1
kind: Ingress
metadata:
name: simple-fanout-example
annotations:
nginx.ingress.kubernetes.io/rewrite-target: /
spec:
rules:
- host: example-ingress.yourfancydomainnamehere.com
http:
paths:
- path: /*
backend:
serviceName: web-example1
servicePort: 8080
- path: /web2/*
backend:
serviceName: web-example2
servicePort: 8080
Make sure you update the host value under the first rule to point to your new DNS record (that fronts your Ingress Controller Load Balancer). i.e. the “example-ingress.yourfancydomainnamehere.com” bit needs to change to your own host / A record you created that points to your own Load Balancer IP address.
The configuration above is a typical “fanout” ingress setup. It provides two rules for two different paths on the host DNS you setup and allows you to route HTTP traffic to different services based on the hostname/path.
This is super useful as you can front multiple different services with a single Load Balancer.
- example-ingress.yourfancydomainnamehere.com/* -> points to your simple web deployment backed by the web-example1 service you exposed it on. Any request that does not match any other rule will be directed to this service (*).
- example-ingress.yourfancydomainnamehere.com/web2/* -> points to your web-example2 service. If you hit your hostname with the path /web2/* the request will go to this service.
Testing
Try browse to the first hostname using your own DNS record and try different combinations that match the rules you defined in your ingress rule on HTTP. You should get the web-example1 “hello-app” being served from your web-example1 pod for any request that does not match /web2/*. E.g. /foo.
For /web2/* you should get the web-example2 “hello-app” default web page. It’ll also display the name of the pod it was served from (in my case web-example2-75fd68f658-f8xcd).
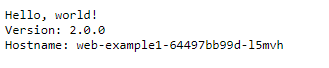
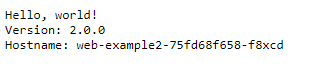
Conclusion
Congratulations! You now have a single Load Balancer fronting an NGINX Ingress Controller on DigitalOcean Kubernetes.
You can now expose multiple Kubernetes run services / deployments from a single Ingress and avoid the need to have multiple Load Balancers running (and costing you money!)